EARLY WARNING SYSTEM PROJECT: LESSONS LEARNT FROM VBAS AND FARMERS.
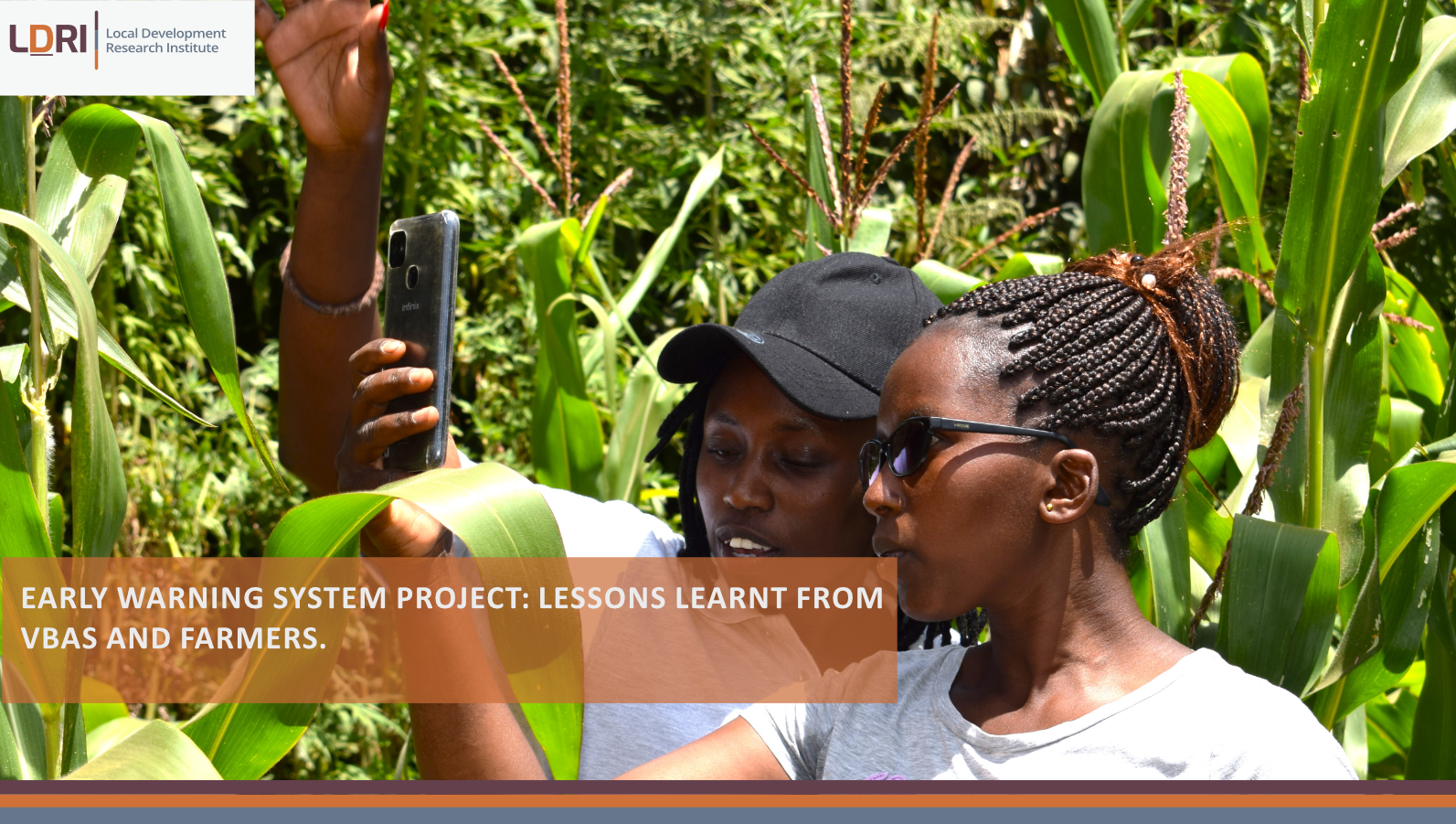
In the past few years (2020 – 2022), Kenya has been experiencing increased and extreme drought tendencies with about 4.2 million people experiencing acute food insecurity. These incidents have forced the majority of the population to leave their homes in search of food and water for both human and animal consumption. (Integrated Food Security Phase Classification (IPC), 2022).
Irregularly distributed and below-average rainfall has led to detrimental effects of declined crop production and lack of pasture coupled with disease outbreaks. This has led to human starvation and a high mortality rate in livestock, especially in the ASAL areas and pastoral communities (ASAL Humanitarian Network (AHN), 2022). In recent years, the use of Artificial Intelligence (AI) to predict weather patterns was one way of averting drought and disease outbreaks.
Farmers rarely trust sources of weather information due to the inconsistency and unreliability of weather predictions. They instead rely on indigenous weather signs such as the shedding of leaves from certain trees, the chirping of certain birds, the migration of birds and insects, and the temperature differences between day and night. In addition, most AI and machine learning technologies give generalized information for a certain region but do not go a step further to give localized information at the village level. Farmers at the grass-root level rarely get warning information on time and if they do, it is not customized for their area but generalized for the whole county or only for arid and semi-arid counties.
This is what LDRI has been working towards, since February 2021, collecting real-time ground-truth data through mobile phones and then comparing it with data/imagery from satellites and remote sensing technology to come up with an effective early warning system that will be useful to farmers. Involving farmers during the implementation of the project was a great way of building trust as farmers felt they owned the project, which increased the accuracy of the data collected. Field agents were identified to assist in data collection. They were selected from all the agroecological zones in the Embu and Kiambu counties. These field agents were given the task of comparing maize and beans performance across the zones, holding other factors, like maize and beans varieties, fertilizer used, pesticides used, soil condition, and amount of rainfall, constant. The zones that were considered were the High altitude zone where Tea was majorly grown, the Mid altitude/ Transitional zone where coffee thrived well, and the low-altitude zone which was the Arid and Semi-Arid lands (ASALs). Different zones had maize varieties suited for those specific areas.
The successful implementation of the Village Based Advisors project motivated LDRI to select VBAs as the field agents to participate in the Early Warning System project. A total of 20 VBAs were identified in Embu and Kiambu Counties. The criteria for selecting the VBAs were based on Age, gender, and proficiency in the use of smartphones. Youth VBAs were preferable as they were tech-savvy and therefore could efficiently operate a smartphone. At least two-thirds of the VBAs were women thus promoting gender inclusivity.
Recap of season 1
During the March-May 2022 planting season, the 20 VBAs were tasked with identifying and selecting 20 farmers from their villages to participate in the project. Thus, a sample of 403 farmers was selected. The criteria used by the VBAs to select the farmers were based on various factors such as distance from VBA’s homestead, farmer relations, location of demo plot, and nature of the farmer in undertaking farming activities. Farmers closer to the VBA were selected to minimize the distance covered when moving from one farmer to the next. Farmers with good relations with the VBA were preferred as it promoted trust. In addition, farmers who were near roads were selected as the location was suitable for establishing demonstration plots, where other farmers could see and motivate them to adopt the varieties grown in those demonstration plots. The march-May 2022 season was riddled with low rainfall, and pest infestation, especially Fall Armyworm which led to low crop yield and total crop failure in the ASALs. Both VBAs and farmers were provided with maize sample seeds of DK 777, DKC 9089, and DKC 8033 varieties from Bayer East Africa seed company. The farmers established demonstration plots and also planted their own-sourced varieties on their main farm. The VBA field agents collected data from the two plots (demo and main farm) established by farmers.
Data collection process
For data collection to be efficient, the VBAs had to undergo training on the use of the ODK application. All the VBAs were tech-savvy and hence could operate a smartphone. Thus, were issued with smartphones and taught how to use the ODK application. information collected from the farmers was as follows;
- Farmer demographics.
This included the name, gender, phone number, age of the farmers, the number of people living in the households, and the number of children under the age of 5 years old. The purpose of this data was to get to know the farmers’ characteristics and child nutrition practices for those with children below 5 years old. Information on the gender and age of the farmers was necessary to ensure women and youth were not excluded from the project. The phone number was a unique identifier as they were specific to each farmer. Although the majority of the farmers had multiple phone numbers in use, the most frequently used number was recorded as the default phone number. The number of people living in the households was essential in determining the food security status of the household while information on children under the age of 5 was imperative in determining the malnutrition status in the household. A study by Heckert et al. (2019) showed that women who were empowered with agricultural skills had a positive impact on their children’s nutritional status.
- Location and mapping data
In addition, data on the location of the farmers was important in mapping where the farms were located through geo-points and geo-shapes. This information enabled the farms to be identified through satellite. The first mapping data collected was on onboarding the farmers and their farms to the project. The VBAs collected location details of where the main farm and the demonstration plots of the farmers were located. However, since farmers are prone to make changes to the locations of these two sites, it was necessary to conduct a second mapping phase, two months after the first onboarding or when the farmers are doing their top-dressing of maize crops. The second mapping was a way of enhancing the accuracy of the geo-points and geo-shapes collected during onboarding. This ensured quality assurance of the data collected.
- Crop production activities
The establishment of demonstration plots was necessary to compare the performance of maize crops from the main farm and the demonstration plot. Data was collected on farmers’ cropping techniques, that is, whether they practiced pure stands or mixed cropping. The most used technique was mixed cropping where legumes such as beans and cowpeas are intercropped with maize. On the other hand, farmers were advised to establish the demonstration plots as pure stands for the maize varieties provided. This was to provide an ideal environment for the hybrid varieties to grow and assess their performance. Additionally, data on the primary and secondary crops were collected in form of pictures. VBAs took pictures of the crops as evidence of their performance. These weekly visits proved efficient as visible changes were seen in how the plant advanced from stage to stage, how the weeding of the farm was conducted, the application of top dressing fertilizer, and even the harvesting of crops. As the VBAs conducted the weekly visits, they also collected information on the farm activities conducted and farm inputs used by the farmers. Data collected on farm activities included planting, weeding, top-dressing, application of pesticides, supplementary irrigation, harvesting, and pest control (non-pesticide practices). Data collected on the farm inputs applied during the visits included fertilizer, pesticide, herbicide, and soil enhancer.
- Recording of audio
Lastly, the VBAs recorded audio on any issues they experienced on the farm while collecting data. The purpose was to ensure that both visual and audio information was collected to increase the accuracy and quality of data. The audios proved very efficient during the March-May 2022 season since information such as theft of crops, crop destruction by both wild and domestic animals, and chopping of maize crops due to crop failure, were recorded. The audio files provided adequate explanations for these situations on the farm, which could have otherwise been undefined outliers. In addition, the farmers felt more involved in the project since their grievances were heard via the audio recording.
Challenges and lessons learned from cohort 1
There were cases where the farmers refused data to be collected on their farms, while in other cases, farmers did not apply GAPs on their farms. In addition, some farmers still did not understand what the project was all about and hence became uncooperative. Instances, where squirrels and monkeys removed seeds from the soil, were common and, in some farms, cows would invade the crops. Heavy rains also hindered data collection. Pest infestation was also a menace, especially Fall Armyworm. In a certain case, the farmers had suggested killing the birds that were removing planted seeds in the soil; however, this would have distorted the natural ecosystem of the area since birds also feed on pests and insects which affect maize and beans.
Constant monitoring of the VBAs as they collected data was imperative to ensure the quality of data. VBAs had to be retrained on the use of ODK as a way of minimizing errors in data collection. Regular communication with VBAs through WhatsApp, SMS, and conference calls was very efficient in getting real-time information on what was happening on the ground.
The second cohort of 20 VBAs was identified and selected to collect data during the October – December 2022 planting season. Although the agroecological regions remained the same as those from the previous season, the areas where data collection took place were different. The importance of selecting new areas with new VBAs was to collect data from a widespread region factoring different environments of VBAs and farmers, enriching the data collected. Lessons learned from the previous cohort of VBAs were effective training of the newly selected group and ensuring seamless collection of data. For instance, the VBAs needed to inform farmers about the project and ensure they understand what it entailed before collecting data from them. Secondly, gender dynamics were skewed towards the female gender due to the nature of women as providers of agricultural labour. It was with this reasoning that the female VBAs in the second cohort were 65% while the male VBAs was 35%.
The March-May 2022 planting season was a disaster because the majority of farmers experienced crop losses and those in ASALs suffered total crop failure.
Constant communication and retraining of VBAs were critical in ensuring VBAs collected the right information. Frequent monitoring of VBAs through field visits by staff guaranteed the quality of data collected. In addition, informing farmers about the project and constant reminders of the data collection aspect of the project promoted cooperation and minimized farmers dropping out.
Reference materials
ASAL Humanitarian Network (AHN). (2022). Drought situation in the Kenya ASAL areas now at crisis level. Published on October 5th, 2022. https://reliefweb.int/report/kenya/drought-situation-kenya-asal-areas-now-crisis-level#:~:text=Nairobi%2C%20Kenya%3B%20October%205th%2C,and%20massive%20displacement%20of%20populations. Retrieved on 23rd November 2022.
Heckert, J., Olney, D.K. and Ruel, M. T. (2019). Is women’s empowerment a pathway to improving child nutrition outcomes in a nutrition-sensitive agriculture program? Evidence from a randomized controlled trial in Burkina Faso. Journal of Social Science & Medicine, 233 (2019) 93–102. https://doi.org/10.1016/j.socscimed.2019.05.016
Integrated Food Security Phase Classification (IPC). (2022). Acute Food Insecurity and Acute Malnutrition Analysis (July – December 2022). Published on September 28, 2022. https://reliefweb.int/report/kenya/kenya-ipc-acute-food-insecurity-and-acute-malnutrition-analysis-july-december-2022-published-september-28-2022#:~:text=According%20to%20the%20most%20recent,IPC%20Phase%204%20(Emergency)